Detecting Corners
Страница в процессе перевода. |
Corner Detection, which is a subset of Interest Point Detection, tries to detect points in the image which have a well-defined position and can be robustly detected in multiple images of the same scene. Very often, these points lie along the corners or edges of objects in the image - hence the name.
Corner detection is useful in several computer vision tasks - such as Image Registration, Motion Detection and Panaroma Stitching. This is because if the locations of the same points are known in two different images, it gives a reference to align those images. Corners, with their well-defined positions serve as good candidates for such points.
using Images, TestImages
img = Gray.(testimage("house"))
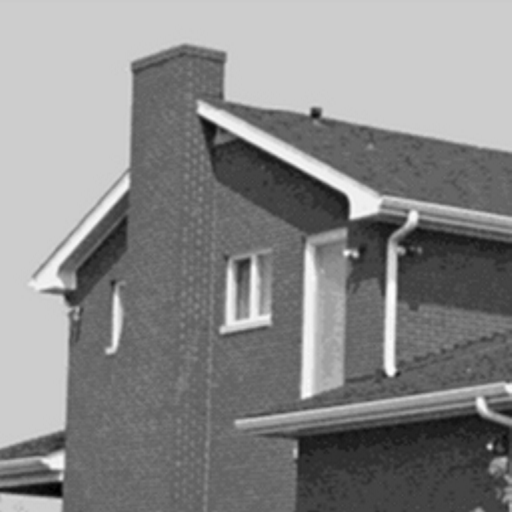
We use this image of a house, with numerous edges and corners.
The imcorner
function can be used to detect corners in the image - and it returns an array of booleans, where a true
value denotes that the corresponding pixel may be a corner. We use this to mark those pixels in red on a copy of the image.
corners = imcorner(img)
img_copy = RGB.(img)
img_copy[corners] .= RGB(1.0, 0.0, 0.0)
img_copy
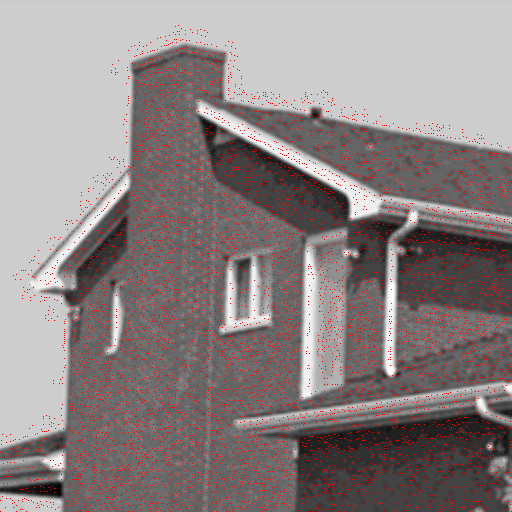
As you can see, several points which lie in the interior of the object (the house) have also been detected as corners. We can fix this by specifying a higher threshold percentile.
corners = imcorner(img, Percentile(98.5))
img_copy2 = RGB.(img)
img_copy2[corners] .= RGB(1.0, 0.0, 0.0)
img_copy2
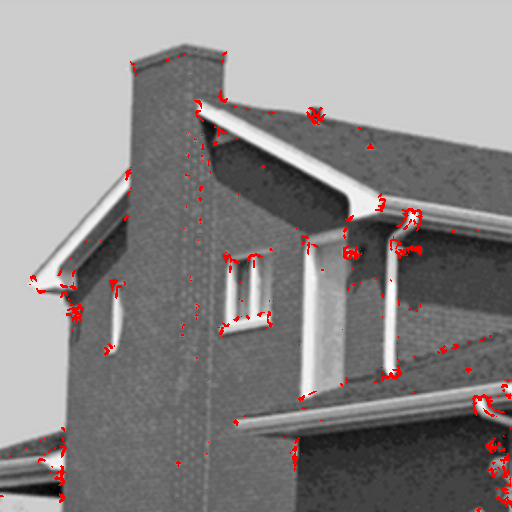
This seems much better. A detailed documentation of the function parameters can be found in the documentation of imcorner
.
Internally, imcorner
uses one of three algorithms: Harris, Shi Tomasi or Kitchen Rosenfield to detect corners. Which one to use can be specified using the method
parameter to imcorner
. Each algorithm also has a separate method.
detection_methods = [harris, shi_tomasi, kitchen_rosenfeld]
img_copies = [RGB.(img) for i in 1:length(detection_methods)]
for i in 1:length(detection_methods)
corners = imcorner(img, Percentile(98.5); method=detection_methods[i])
img_copies[i] = RGB.(img)
img_copies[i][corners] .= RGB(1.0, 0.0, 0.0)
end
mosaicview(img_copies; nrow=1)
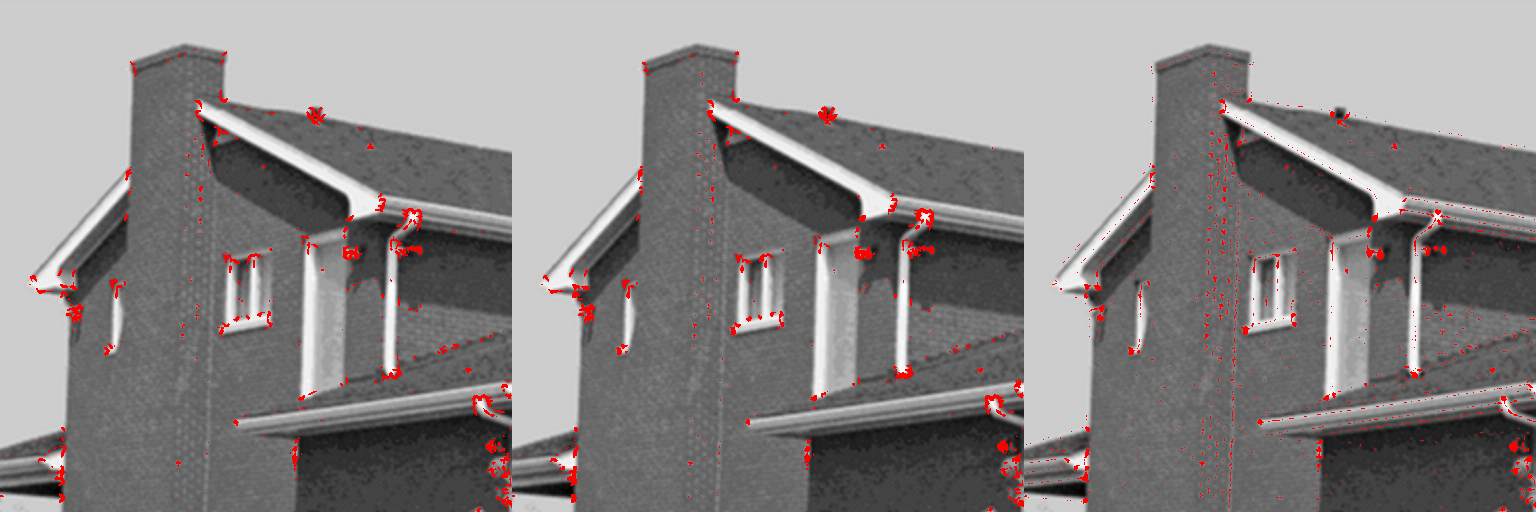
These algorithms use the gradient of the image to identify corners, because intensities change abruptly at corner points, giving rise to large gradients. However, this makes them computationally expensive.
The FAST (Features from Accelerated Segment Test) Corner Detector is a feature detection algorithm which is designed to be computationally cheaper, and hence much faster. It classifies a pixel as a corner if at least contiguous points out of the 16 points in a circle around it have intensities either higher (or lower) than that of by a certain threshold .
corners = fastcorners(img, 11, 0.1) # fastcorners(img, n, t) where n and t are optional
img_copy3 = RGB.(img)
img_copy3[corners] .= RGB(1.0, 0.0, 0.0)
img_copy3
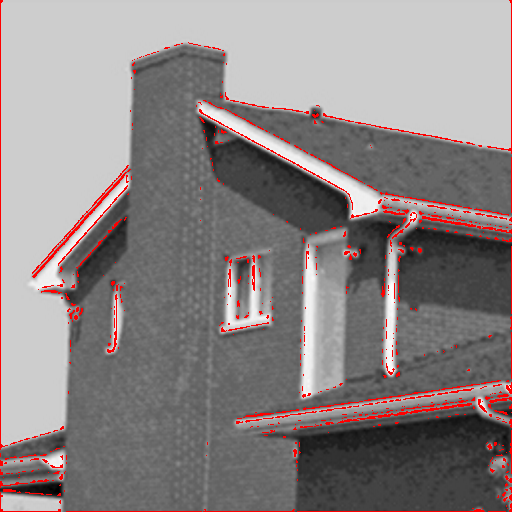
This page was generated using DemoCards.jl and Literate.jl.